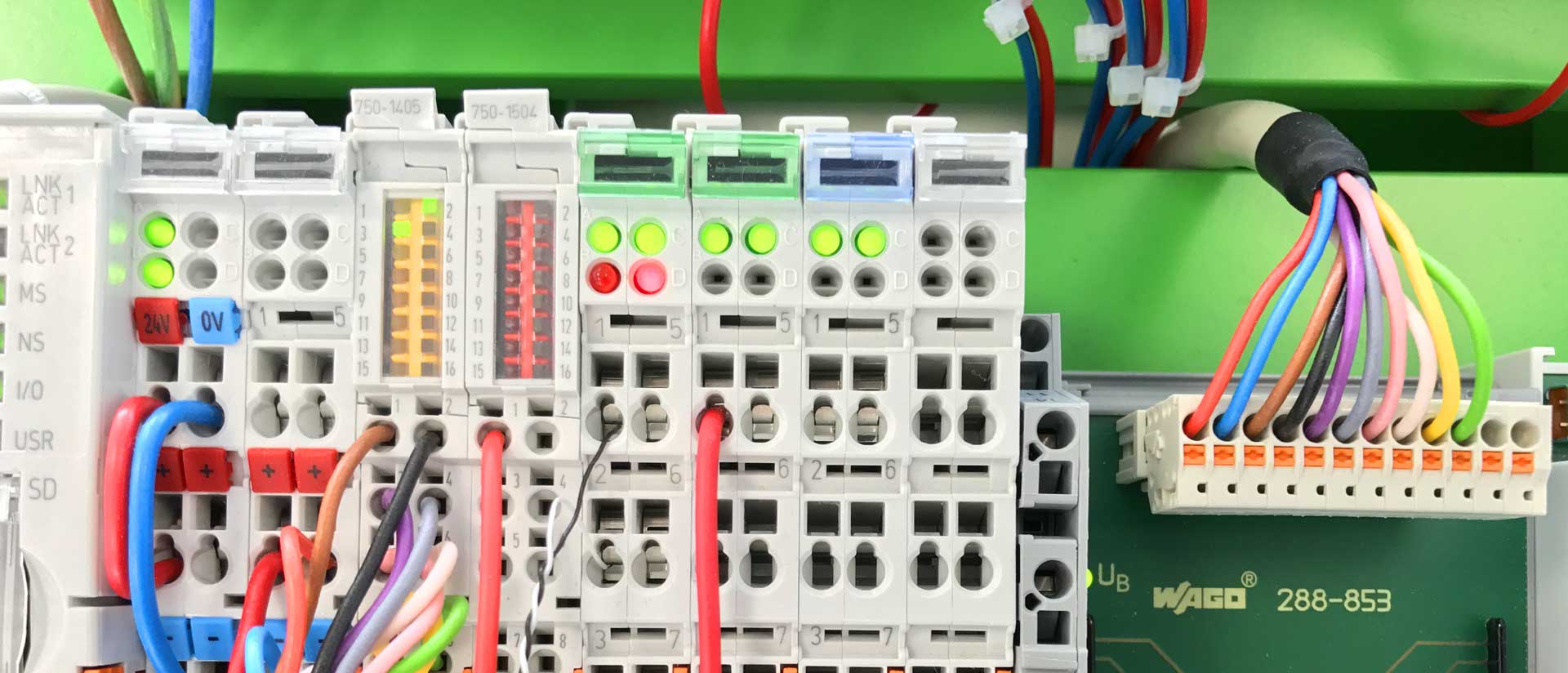
Zukunftsmusik
How automation will affect the future of work in Germany
By Laura D’Andrea Tyson
I. Predictions Based on History, Theory, and Current Research
Intelligent machines are transforming the way we produce, the way we work, the way we learn, and the way we live throughout the world. Individual drivers and major logistics companies such as UPS are using new technologies to optimize their route planning. Companies such as BMW and Tesla have already released self-driving features in their automobiles, which are produced with the help of sophisticated robots. DHL has launched a fully automated and intelligent urban drone-delivery system in China. Drones are also being used to deliver health supplies to remote locations in the poorest countries. The Associated Press is using artificial intelligence to help write news stories. 3-D printers are being used to produce replacement parts—for both machines and humans. There is a major German business initiative spearheaded by BMW to increase the use of 3-D printing in the automotive industry. AT&T has a broad worker-training program and, in collaboration with Udacity, is offering online nanodegrees in data analytics and other digital tools. Siemens is one of the largest providers of vocational training in Germany, with a focus on topics such as data analytics, software development, and data security.
Marvelous new technologies that automate work promise higher productivity, greater efficiency, more safety, flexibility, and convenience. But they are also stoking fears about their effects on jobs, skills, and wages. A 2019 survey by the Pew Research Center found that 48% of Americans think that automation has mostly hurt workers, and 76% believe that inequality will increase if robots and computers perform most of the jobs currently being done by humans. In the EU, according to a 2017 survey published by the European Commission, 74% of citizens expect that robots and AI will destroy more jobs than they create. The primary concerns among workers and citizens are about both the quantity and the quality of jobs. Will there be enough jobs for workers seeking employment? Will the available jobs have wages that provide meaningful livelihoods for workers and their families? What can companies and policymakers do to alleviate the costs and share the benefits of the new technologies broadly?
Feeding these concerns are several recent studies finding that large shares of employment in both developing and developed countries are “susceptible” to automation using currently available technologies. Both history and economic theory, however, indicate that fears about technological unemployment, a term coined by John Maynard Keynes, nearly a century ago, are misplaced. Historical evidence shows that technological change fuels productivity gains, which in turn fuel income gains, boosting both the demand for goods and services and the demand for human labor to produce them. At least to date, technological progress has not resulted in less employment, but rather in changes in the size and growth of industries, sectors, and occupations.
The assumption that all technologies increase labor demand simply because they raise productivity, however, is wrong. It is possible that some automation technologies may, in fact, reduce total employment because they cause sizable displacement effects but only modest productivity gains. Moreover, even if future automation does not lead to technological unemployment in the short to medium run—which could span decades and the entire lifetimes of workers—labor-saving automation is likely to trigger significant changes in the sectors, skill-mix, and location of jobs, displacing tens of millions of workers around the world and requiring them to transition to new employment opportunities. And if the workers displaced by automation are unable to acquire the skills necessary for new jobs or to find them quickly, both frictional and structural unemployment are likely to increase, causing adverse economy-wide effects on growth and potential output.
Even if future automation does not lead to technological unemployment in the short to medium run—which could span decades and the entire lifetimes of workers—labor-saving automation is likely to trigger significant changes in the sectors, skill-mix, and location of jobs, displacing tens of millions of workers around the world and requiring them to transition to new employment opportunities.
History is replete with periods of productivity enhancing technological change that produce serious social and economic strains. Disruptive technologies can generate huge profits and productivity, but they can also destroy businesses, communities, and individuals, and depress wages. During the Industrial Revolution in England in the nineteenth century, average real wages stagnated for forty years, even as productivity soared. During the last forty years, skill-biased and labor-saving technological progress has eroded middle-skill, middle-wage jobs, polarized the labor market, and exacerbated rising income-inequality in a variety of ways. It has favored more-skilled over less-skilled workers; it has increased capital returns over labor; it has enabled or “turbo-charged” globalization, reducing employment and constrained wage growth for middle-income workers, particularly in manufacturing and tradable services, in developed countries; it has increased the advantages of superstars and the super-lucky; and it has generated rents in highly imperfect markets.
The tide of automation does not automatically lift all boats. Because of its displacement effect, labor-saving automation need not fuel wage increases commensurate with productivity growth. By replacing labor with machines in production tasks and putting downward pressure on wages, automation can reduce labor’s share of value-added (and national) income and increase income inequality. Indeed, there are now several studies indicating that automation has played a major role in decoupling productivity growth and wage growth and is a significant factor behind the decline in the labor share of national income in advanced economies. According to a 2017 study by the International Monetary Fund (IMF), for example, technological progress explains about half of this decline since 1990.
There is a wide range of estimates of the likely speed and scale of disruption of labor markets that will be caused by future automation. Automation, as measured in these studies, encompasses a variety of currently available tools, including computer software, digital platforms, intelligent robots, and machines and systems to carry out tasks that could otherwise be done by human workers. Using a variety of time periods and measurement techniques, existing research concludes that automation’s effects on the future of work and labor markets will be substantial. The University of Oxford’s Carl Benedikt Frey and Michael A. Osborne, for example, found in 2013 that 47% of US occupations and, according to their 2016 study with Citibank, on average 57% of occupations in the 36 countries in the Organization for Economic Cooperation and Development (OECD) are at risk of automation over the next two decades. A study published earlier this year by the OECD finds substantially smaller effects, concluding that 14% of jobs in the OECD have a high risk of automation, 70% or higher, while 32% of jobs have a moderate risk, between 50% and 70%. Moreover, there are notable differences among the OECD countries—ranging from 6% of jobs at high risk of automation in Norway to 34% in the Slovak Republic and 18% in Germany.
A comprehensive January 2017 study by the McKinsey Global Institute (MGI), covering 46 countries and 80% of the global labor force, estimates that while less than 5% of occupations could be fully automated with currently demonstrated technologies, 60% of occupations have 30% or more of their constituent activities that could be automated. A December 2017 MGI report finds that under a likely midpoint scenario for the pace of automation about 15% of the global workforce—or 400 million workers—could be displaced between 2016 and 2030, requiring between 75 and 375 million workers, or 3% to 14% of the global workforce, to change occupational categories. In developed economies, where high labor costs are likely to accelerate the adoption of labor-saving technologies, the share of the workforce that may have to change occupational categories and the skills associated with them is even higher. In these countries, routine jobs in major occupational categories such as production and office support, and jobs requiring a high school education or less are projected to decline, while jobs in occupational categories such as healthcare and care providers, educators, builders, managers, and jobs requiring a college or advanced degree are projected to grow. Automation risks are greatest for routine cognitive tasks like data-collection and data-processing, and routine manual and physical tasks in structured, predictable environments, like production-line jobs in manufacturing. In advanced economies, including the US and European countries, large shares of middle-skill, middle-wage employment consist of such routine jobs.
Automation risks are greatest for routine cognitive tasks like data-collection and data-processing, and routine manual and physical tasks in structured, predictable environments, like production-line jobs in manufacturing.
Existing research also finds a negative correlation between the skill levels and wages of occupations/tasks and their potential for automation. In developed economies, automation is likely to reduce the demand for low- and middle-wage routine jobs, with workers in middle-skill jobs the most vulnerable. Low-wage workers could be somewhat less at risk, since their wages are too low to justify the cost of implementing labor-saving technologies. Middle-wage workers, in contrast, may have high enough earnings to make such technologies cost effective.
These conclusions are evidence of the skill-biased nature of automation — on balance, it reduces the demand for low and middle-skill workers in routine jobs while increasing the demand for high-skill workers performing abstract tasks that require technical and problem-solving skills. The skill-biased nature of technological change has been apparent in the advanced economies over the last several decades and is likely to persist and intensify in the future. The resulting polarization of the labor market is reflected in both widening educational wage-differentials and widening overall wage inequality. As a result of these trends, income inequality is likely to continue to grow in the US and other developed countries as automation continues to substitute for humans in many routine middle-skill and middle-wage occupations, while increasing the demand for workers with the higher skills and education complementary to and required by the new technologies.
II. The Future of Work in Germany
Despite Germany’s high cost of labor, its exposure to labor-saving and skill-biased technological change, and significant automation, the country’s economy is at full employment, the labor force participation rate is relatively high, and wages are growing. Although employment in manufacturing declined significantly between 1970 and 2013, from 40% to 19% of total employment , Germany has the highest share of employment in manufacturing among the advanced economies, twice the share of the UK and the US, and higher than the share in Japan. Germany is also the most automated country in Europe in terms of industrial robots: for every 10,000 workers in the manufacturing industry there are 322 industrial robots, more than three times the European average of 106 industrial robots.
Recent research on the deployment of robots in Germany and US yields important comparative results, revealing significant differences. A 2018 German study found that from 1994 to 2014 an additional industrial robot (defined broadly as an autonomous, automatically controlled, reprogrammable, and multipurpose machine) per 1000 employees directly reduced manufacturing employment by two jobs, but indirectly increased employment in the service sector by approximately the same number, yielding a zero net-effect on total employment in local labor markets specializing in industries with high robot usage. Overall, robots were responsible for 23% of the decline in manufacturing jobs in Germany during this period, driven by a reduction in the number of manufacturing jobs available for new, young labor-market entrants over time. Interestingly, robot adoption did not increase the risk of displacement for incumbent manufacturing workers, suggesting that businesses deployed robots to complement the skills of their existing workers and reshape their tasks rather than to substitute for them. The study finds evidence that these workers were willing to trade-off job stability for lower wage-growth. As a consequence, over time, workers more exposed to robots had lower labor earnings, and automation caused labor shares in exposed regions to decline. The study also shows that while there were positive earnings effects from automation for managers and skilled technical occupations, there were negative earnings effects for the bulk of medium-skilled and low-skilled workers whose routine tasks could be more easily replaced by robots.
Using a similar methodology, a comparable 2017 study, by Daron Acemoglu and Pascual Restrepo, found that from 1990 to 2007 the infiltration of industrial robots undermined both employment and wages in local US labor markets. One additional robot per 1000 employees reduced employment by three to six workers, and wages declined between 0.25 and 0.5 percent. In contrast to the German results, the introduction of robots did not indirectly increase employment in business service or other jobs, so the net effect on employment was negative. Similar to the German results, the negative employment and wage effects were largest for workers with a high-school degree or less and were concentrated in the bottom half of the wage distribution, driving up wage inequality as measured by the 90-10 wage differential.
Assuming a moderate pace of future automation, MGI estimates that, given trendline GDP growth and the projected decline in the working-age population, that there will be enough net jobs created to maintain full employment in Germany through 2030. Nearly a third of the workforce, however, may need to switch occupational groups, causing significant dislocation and transition costs. But employment and wage polarization trends are likely to continue. The share of employment in the bottom two-thirds of the wage distribution is likely to fall, while the share in the top one-third is likely to increase. Moreover, the demand for workers with less than a secondary education is likely to fall, while the demand for workers with a college or more advanced degree is likely to increase. Finally, thousands of workers will need to upskill or reskill to meet the requirements of new job opportunities.
For many German workers currently employed in routine cognitive and physical jobs most likely to succumb to automation, the quality of new job opportunities, as measured by wages and benefits, may be lower than the quality of the old jobs that are displaced. In short, in the absence of offsetting policies, Germany, like other developed countries, may not be headed to a “jobless” future, with high technological unemployment, but to a “good jobless” future, in which a growing share of workers in middle-skill jobs can no longer earn middle-class incomes and sustain middle-class livelihoods.
III. Government, Business, and Labor Responses to the Challenges and Opportunities of Automation
Intelligent machines that can substitute for human labor are creating similar challenges for advanced countries, but governments and businesses in individual countries are responding differently. What can policymakers do to speed and ease the significant displacements and transitions between jobs and occupations that will be triggered by automation? Fiscal and monetary policies to sustain full-employment levels of aggregate demand over the business cycle are critical. As the recent performance of the US, Germany, and many other advanced countries confirms, supportive macro-policies can foster high overall levels of employment and low unemployment levels, even as automation changes the composition of jobs. And over the medium term, policies to promote investment in infrastructure, housing, alternative energy, healthcare, and care for the young and the aging can boost economic competitiveness and inclusive growth while creating additional jobs in occupations that are likely to be augmented rather than displaced by automation.
A second critical policy response is the expansion and redesign of workforce training programs to upskill, reskill, and relocate workers. Unfortunately, most of the advanced countries are unprepared for a faster pace of job reallocation—the destruction and creation of jobs with different skills, in different firms, in different industries, and in different places. Over the past two decades, government outlays for labor-force training and labor-market adjustments have fallen in most OECD countries. Despite evidence of their effectiveness, active labor-market policies in the form of government employment services, job-search assistance, training, and unemployment benefits have been cut in many countries. In the majority of OECD countries, fewer than one-third of jobseekers receive unemployment benefits. In Germany, unemployment insurance is only available after a full year of contributions, and the pension system penalizes career interruptions with substantial reductions in entitlements. In the absence of policies to reallocate workers from disappearing jobs to new ones, automation will result in higher structural and frictional unemployment.
These trends must be reversed. As part of a new social contract for the age of automation, lifelong learning, supported by active labor-market policies, needs to become a reality. As machines take over routine tasks, jobs will change, and work activities that complement intelligent machines will require different skills and higher levels of education. High-level cognitive abilities and complex social interaction skills such as logical reasoning, stronger communication skills, and enhanced social and emotional skills will become more important, while machines take over routine capabilities that are common in the workplace today. The good news is that the demand for workers with the requisite skills is projected to increase, and many routine middle-skilled jobs will be replaced by higher-skill, better paying ones. The challenges for both individual workers and for the societies in which they live, however, are how to equip workers with the required skills and education, as well as how to pay for the sizeable investments in training, education, and other active labor market policies that will be required.
The good news is that the demand for workers with the requisite skills is projected to increase, and many routine middle-skilled jobs will be replaced by higher-skill, better paying ones.
For mid-career workers—with children, mortgages, and other financial responsibilities—training that is measured in weeks and months, not in years, will be necessary, and workers will need financial support to undertake such training. Sending people out for new, lengthy degree-programs at their own expense is not the answer. Instead, “nanodegrees” and stackable credentials, often obtained online and with the help of government training-funds, are likely to gain in importance. Active labor-market policies to promote the reskilling and re-employment of displaced workers are essential, and Germany has made significant strides. German policies include vouchers for individual workers for both job placement services and job training. Private job-placement services eligible for voucher support must have proven track-records in placing individuals in jobs, and voucher payment is conditional on worker tenure in new jobs for at least three months. Germany’s job training voucher system has been shown to increase employment probability for individual workers by two percentage points. Unfortunately, both in Germany and throughout the OECD, workers with lower skills who are most at risk from automation are less likely to take advantage of training and education opportunities. In Germany, the gap in training participation between high- and low-skill adults is the highest in the OECD at approximately 50 percentage points, compared to 43 percentage points in the US.
As a result of the displacements and transitions caused by automation, a growing number of workers are likely to find themselves forced to change jobs, occupations, employers, and even locations of work frequently, and they may find themselves working in various forms of “non-standard employment,” including self-employment, temporary employment, contract employment, and independent-contractor employment with platform businesses like Uber. Governments will have to develop and finance policies to provide universal and portable social benefits like healthcare, childcare, and retirement security to workers in non-standard employment. Recent studies by the International Labor Organization (ILO) and the OECD provide detailed recommendations for the provision of such social protections. For employees, portability of traditional social protections between EU member states already exists under Regulations (EC) 883/2004 and 987/2009. But there is no assurance of portability of benefits across employers within a country, nor is there assurance of the portability across different types of work—for example, between traditional employment and self-employment.
Ultimately, decisions to deploy automation technologies are made not by policymakers but by businesses responding to incentives that are heavily affected by policies, including tax policy. A relatively high cost of labor, including taxes on labor, increases business incentives to adopt labor-saving technology. So do taxes that reduce the cost of capital through accelerated amortization, interest deductions, and other mechanisms. Indeed, economists Daron Acemoglu and Pascual Restrepo suggest in a 2019 paper for the Journal of Economic Perspectives, “Automation and New Tasks: How Technology Displaces and Reinstates Labor,” that the current composition of taxes in the US and other developed countries is accelerating the adoption of labor-saving automation. And the more the labor-saving automation paradigm is adopted as a competitive strategy, the more market incentives will favor investing in such automation at the expense of other strategies that focus on creating new labor-intensive tasks rather than substituting for labor.
Business decisions are also influenced by the institutions representing workers and their interests. Collective bargaining can bring workers’ voices into decisions around technology adoption, deployment, and adjustment processes. Unfortunately, worker voices have become more muted in the last several decades, as the share of workers who are members of unions and covered by collective agreements has declined sharply throughout the OECD, including Germany. Today, the German share is about 17%, compared to 10.5% in the US. There are also several unique institutional features of German business governance—works councils, supervisory councils, and national unions and employers’ associations organized by sector—which are likely to affect company decisions about the pace and magnitude of automation and its effects on employment levels, wages, hours of work, and other working conditions. Small- and medium-sized businesses, often privately or family owned, are also likely to shape such decisions in Germany in ways that give greater voice to workers and local communities and that assess the costs and benefits of labor-saving automation strategies differently.
There are also several unique institutional features of German business governance—works councils, supervisory councils, and national unions and employers’ associations organized by sector—which are likely to affect company decisions about the pace and magnitude of automation and its effects on employment levels, wages, hours of work, and other working conditions.
The effects of automation on the future of work are not technologically determined. They can be shaped by the policies and actions taken by governments, businesses, workers, and citizens. Recent studies by the ILO and the OECD suggest guidelines for inclusive strategies to share the costs and benefits of automation broadly. Germany, building on its strong social contract and labor-market institutions, is developing an ambitious agenda to realize this goal while maintaining its strong global competitiveness. The US and other OECD countries can learn from Germany’s forward-looking vision and leadership.
How will policymakers respond to the transition costs and income inequalities resulting from the coming wave of automation? Who will bear the dislocation costs on the path to an automated future? Will the benefits of intelligent machines be widely shared or be captured by a small percentage of the population? The answers to these questions depend not on the design of these machines but on the design of intelligent policies and intelligent business practices to reap their benefits and share them broadly across society.